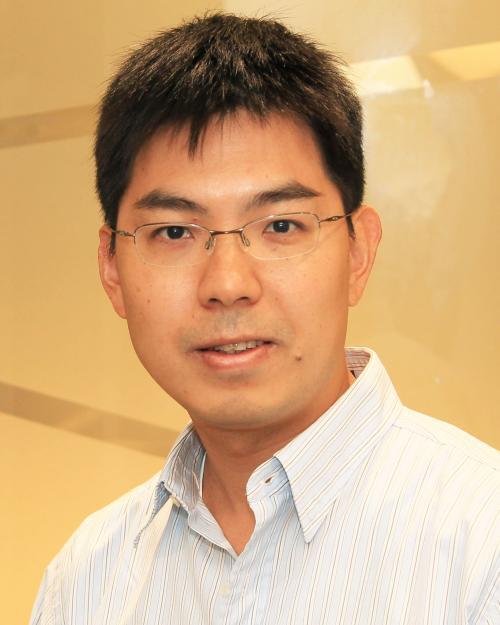
Going beyond generic deep learning for wireless communications
Presentation Menu
In recent years, deep learning has become an essential component of wireless communication design. However, most attempts simply utilize generic modules such as Multi-Layer Perceptron (MLP), Convolutional Neural Network (CNN), and others. While these generic designs have proven to be somewhat effective in experiments, they may not be the ideal fit for wireless communications. This presentation argues that domain knowledge in wireless communications should be leveraged when designing deep learning algorithms for wireless applications. We will explore three examples of how this domain knowledge can be incorporated. The first one involves deep unfolding of an optimization algorithm to guide the deep neural network architecture. The second one employs a Graph Neural Network to mimic the network topology of multiple users and multiple base stations. The third one is the use of complex-valued neural networks to match the characteristics of wireless communication signals. Experimental studies reveal the benefits of using deep learning with structures specifically tailored for wireless applications.