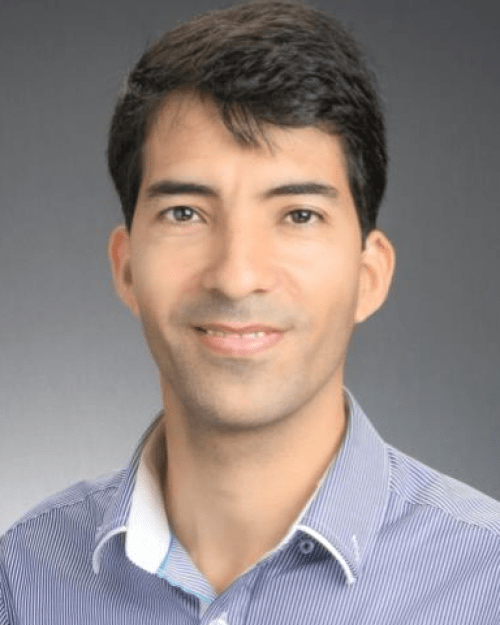
Interference-Aware End-to-End Communications: A Road to AI-Native Air Interface
Presentation Menu
The transition to AI-native radio access networks (RAN) demands a fundamental rethinking of the air interface, particularly in interference-limited environments. This talk explores interference-aware end-to-end communication as a critical pathway toward truly AI-native wireless systems. We present how emerging deep learning techniques—from autoencoder-based physical layer designs to reinforcement learning-driven resource allocation—can jointly optimize spectral efficiency, reliability, and scalability while autonomously mitigating interference. The discussion covers: (1) novel neural network architectures that replace traditional interference management modules with adaptive, context-aware solutions; (2) integration of these approaches with 5G-Advanced/6G standards; and (3) practical challenges in deployment, including real-time inference latency and generalization across diverse network conditions.